Emerging technologies in financial services - The AI Story
- Ampersand Partners
- Mar 16, 2021
- 8 min read
Updated: Oct 31, 2023

Although advancements in artificial intelligence (AI) and related technologies are transforming financial services firms, there is still ambiguity at executive levels as to exactly what the terms mean and how they may be applicable in the real world. This blog demystifies some of the fundamental concepts of AI and posits a diagnostic approach to enable organisations to evaluate potential impact.
The AI Growth Story
Over the last few years, new technologies powered by artificial intelligence (AI) and machine learning (ML) have radically altered the landscape both visibly such as in the area of different consumer propositions and less visibly including in the operational models used by firms. This took longer than might have been expected and firms initially needed to build bespoke R&D arms to utilise these tools, however, over the last few years, the pace of change has increased as these tools and techniques have become much more accessible.
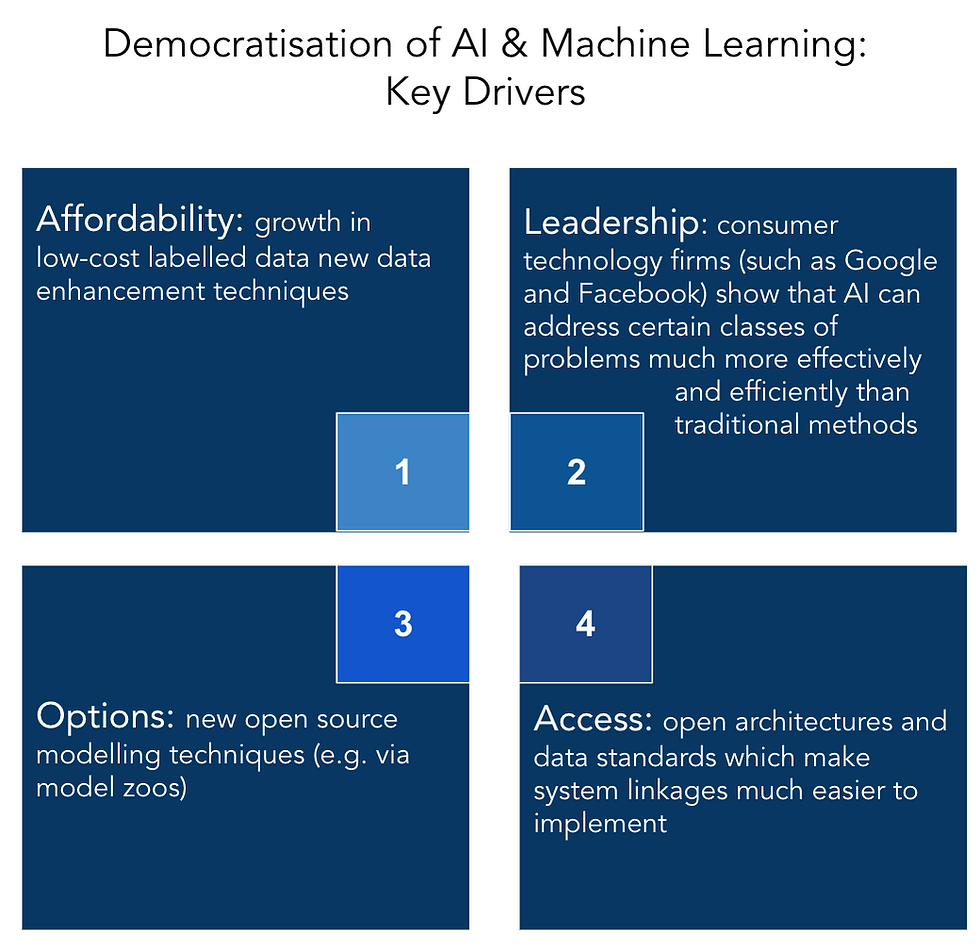
Missed Opportunities
Consumer financial services and insurance have lagged the tech and consumer retail industries in exploiting these developments, however, even in these sectors some technologies are becoming well-understood and exploited including open architecture systems, API connectivity (due to the regulatory impact of Open Banking) and installations in the cloud. For some other emerging technologies, although the intellectual case is clear, the applicability of those to real-world issues has proven to be more limited – a prime example of this is blockchain which has struggled to find yet effective scale use-cases beyond crypto-assets.
What actually constitutes AI?
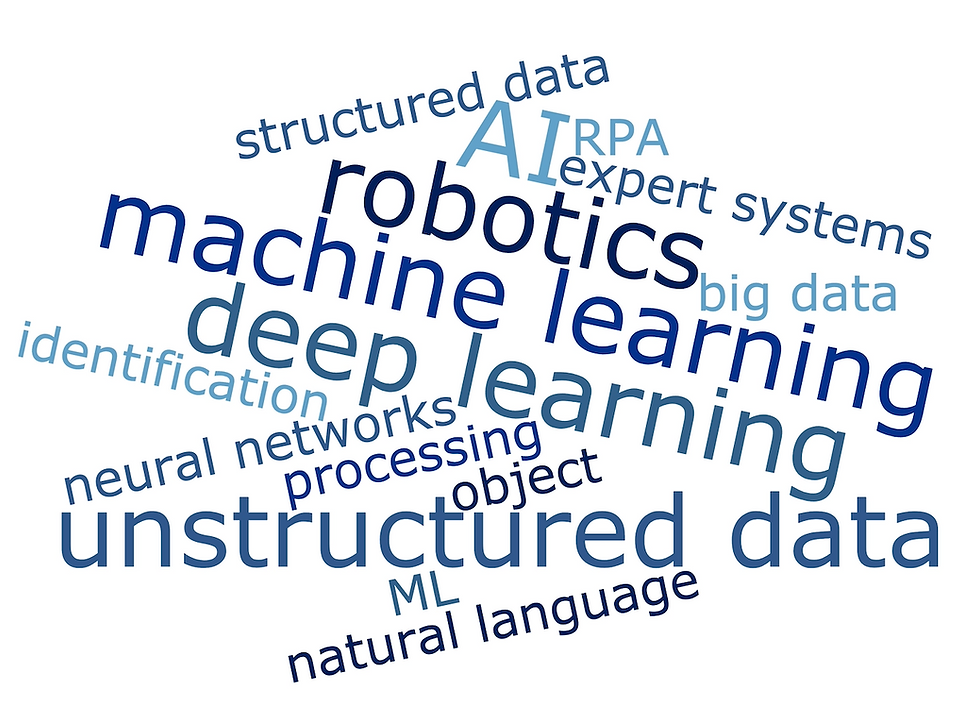
There are many and varied definitions of AI and the term is often interchangeably used with machine learning (ML). The term AI broadly applies to the decision making and analytical capabilities which assumes a degree of human-like intelligence. ML is a specific application or key field of study of AI that uses algorithms for the analysis, manipulation, pattern recognition and prediction of data. Artificial neural networks used in ML are sophisticated models which have been inspired by communication nodes and information processing in biological systems, principally the human brain. Neural networks with multiple layers (called deep learning), are currently at the cutting edge of ML research, and solve complex problems such as object identification, digital vision and natural language processing (NLP) with increasingly high degrees of accuracy and at very high speeds unachievable by manual methods. Expert systems are systems which emulate human decision making – these have traditionally been based on “if-then” rules, but the advent of AI has meant that automatic inferences can be drawn from the accessible knowledge base.
Big data is a collective name that refers to the use of very large datasets for ML applications. It covers both structured and unstructured forms of data from internal and external sources. Structured data is highly organised, and can be codified, sorted and searched in files. Although structured data can be analysed with traditional computing techniques (such as via relational databases), the sheer amount of data today provides an operational challenge. Unstructured data, on the other hand, typically has no predefined form and can include snippets of text, video and other environmental inputs and inferences. The individual data points may have no clear relationship with each other which makes it difficult to capture and organise the data. Specific forms of ML facilitate the process of interpreting unstructured data. For instance, NLP is a branch of ML that facilitates the interactions between computers and human languages. This technology allows the identification, organisation and analysis of large amounts of unstructured data from written or oral language inputs and processes them with decision analytics, to produce outputs often in the form of quantitative metrics such as scores.
Robotics technology applies broadly to the application of physical, autonomous agents that show characteristics of behaving intelligently. This field encompasses areas of ML as well as drawing heavily from other engineering disciplines. It has been applied across many industries to the standardisation and automation of front-to-back processes through the concept of robotic process automation (RPA) which is a business process automation technology based on the notion of metaphorical software robots as ML workers. In many cases, the existing operating model can be kept intact while introducing automation – this therefore can allow quicker implementation than change dependent on re-engineering. RPA and similar techniques have now become an important contributor to the efficiency initiatives that have been pursued by many firms, including financial institutions.
What types of problems do these technologies help resolve?
When applied in financial services, there are a variety of benefits which have been seen by participants. These can be categorised broadly into two categories:
Augmented decision making:
Using AI tools and techniques can lead to an improvement in both the quality and consistency of decision making. Predictive analytics can allow a wider range of variables to be taken into account and an inherent feedback loop can be used to ensure continuous improvement. Value has also been demonstrated in the areas of compliance and risk management where the auditability and consistency of decisions can be made much clearer and techniques can be used to uncover hitherto unrecognised instances of risk or compliance breach.
Performance improvement:
Using AI and ML, firms have been able to both increase speed and straight through processing — this is particularly of relevance where there are discordant breaks in customer journeys involving multiple handoffs. In addition, error rates can be mitigated quickly particularly for repetitive tasks which require high degrees of accuracy. In addition to improvements in customer satisfaction, this can also drive a significantly improved cost profile both due to the reduction of manual inputs but also from the consequent improvement in accuracy leading to lower rework.
Potential for application of these technologies in financial services
AI and ML are helping to fundamentally transform the operating model of banks, insurers and other financial institutions. Augmented decision making is being applied in the front office and provides insights as to customer interactions and behaviour to enable better risk underwriting while improving performance through automation such as in the area of on-boarding and KYC. RPA has further streamlined operating platforms in the back-office, and compliance & surveillance functions in the middle office. The ability to increase specificity as a result of these technologies has in turn allowed banks to augment their value proposition through specialised offerings across their target client segments.
Although individual strategies, target customer segments and markets will impact the specific applicability of this to each entity, some areas of interest are likely to include:
Client interactions and experience:
Clients and prospects can interact with their financial institution24/7 through the support of chatbots that handle online customer queries. Customer contact is increasingly online with an immediate response time and feedback loop, replacing traditional call centres and branch structures. Client experience has increased dramatically through a wider and more specific access to relevant information on banks’ websites and client applications. The client is free to select his/her own service time independent of opening hour constraints.
Onboarding:
NLP facilitates document recognition and user identification. Clients upload their personal data to central depositories with minimal manual interactions by staff members. Standard documents such as passports and driver licenses are recognised and validated against publicly available data sources. This technology application has provided more data accuracy and less errors in capturing the relevant data. Recent examples at challenger banks (e.g. Revolut) illustrate though that manual oversight remains still an important part of the compliance process.
Credit underwriting and risk assessment:
Behavioural data, using ML, neural network and other advanced statistical techniques have added new analytical tools to the bank’s underwriting process. Underwriting processes using new sources of structured and unstructured data (particularly in “thin file” cases) and propensity modelling based on pattern recognition can estimate default probability more accurately than traditional methods which are heavily reliant on credit scoring or manual decisioning. Using AI allows detection of anomalies and unearthing of complex patterns that may flag potentially troubling behaviour. Alternative data sources such as applicants’ payment history, shopping behaviour, management information system and public comments can be integrated in the credit decision. Neo-banks such as OakNorth and lending platforms such as Iwoca have even made these services available as a licencing product for other financial institutions. From a regulatory perspective, some of these tools have also been helpful in determining affordability and hence driving responsible lending.
Research and analytics:
Large parts of financial institution research processes are rule-based and standardised in accordance with defined analytical frameworks and quantitative models. In the new world, documents are read by ML algorithms to extract important data and visualise information for readability. ML applications can further create models autonomously after observing the unstructured data and back-testing to learn from previous mistakes to improve accuracy. This step is often referred to as contextualising and has made a substantial way forward with the emerging NLP technologies. NLP models can provide deep insights in the news flow of traditional media, social media and other relevant publications from research departments, corporations and government authorities. Kensho, now owned by S&P Global, started to cooperate early with several financial institutions in applying ML and analytics systems in particular to research through document scanning for key words and solving early stage analytical problems.
Risk and capital management:
ML and predictive analytics through specific algorithms have further advanced the application of methodologies such as Value-at-Risk (VaR) to assess and manage risk. In particular, they can support the automation of crucial functions across compliance, trade surveillance and fraud detection. There are several providers in this space with specific services from cyber security such as Aponix and regulatory compliance such as Cordium and Throgmorton. Several quantitative asset managers have been at the forefront to develop AI solutions and integrate more complex forms of alternative data in their risk management frameworks. These solutions get increasingly applied as integrated treasury and risk management solutions.
Performance improvement and operational efficiency:
RPA has become an important contributor to the cost cutting initiatives that have been pursued by many financial institutions. In a typical implementation, RPA develops the action list by watching the user perform that task in the application's graphical user interface (GUI), and then performs the automation by repeating those tasks directly in the GUI, often with its own login that mimics a human user. This means that little change is required to systems – in fact, RPA has been proved to be very useful in situations where multiple legacy systems are involved and revamping them is not economically justifiable. Large global banks such as Deutsche Bank, Barclays and Citigroup are using RPA as a core tool in their ongoing cost reduction initiatives
Culture and leadership:
Ways of working have dramatically changed due to the COVID 19 impact, driving significant drops in employee engagement at most financial institutions. The application of ML can help perform culture diagnoses and boost people engagement through targeted change plans. Concrete steps seen include diagnosing organisational culture using advanced data science with intelligent surveys (based on nudge theory), analysing organisational sentiment through unstructured data, improving culture by deploying machine learning driven insights and monitoring and assuring the effectiveness of the changes in near real-time.
How should firms approach this
We suggest a well-structured four phase plan to getting to grips with this – this is illustrated below. However, it is critical to note that this is not a “one-and-done” activity – AI/ML possibilities and capabilities develop rapidly and in different directions as a result, it is more important to have relatively short term aims and then recalibrate on a regular basis. As a result, a test-and-learn approach to each of these would be most appropriate– we would suggest running a Proof-of-Concept trial, to identify if this would be worth taking forward and in which directions.

Example Diagnostic template of phase 1

Conclusion
Although it is becoming clear across many C-suites and Boards that AI/ML has the ability to transform business, many firms struggle to deal with this in a structured way and instead focus on individual initiatives rather than taking an overall perspective. In our view, to benefit from this, organisations will need to take a holistic view of where this is applicable and then implement a structured programme to build this within a flexible, agile framework.
It may be the case that external support would be helpful to support this effort - at Ampersand Partners, we combine sector expertise with a practical knowledge of these technologies and are able to offer unbiased support to clients who are looking to understand how to best move ahead in this exciting and rapidly changing area.
Look out for upcoming blogs on the rise of other emerging technologies and their relevance to financial services.
Ampersand Partners is a management consultancy, whose Finance and Insurance practice helps financial services and insurance sectors harness the power of technology to navigate their fast changing market environment.
Please reach out to the authors if you are interested in exploring how artificial intelligence can help transform your organisation.
Kelvin Malayapillay
kelvin@ampersand.partners
Joerg Ruetschi
joerg@ampersand.partners
Comments